Examples Of Using Fairlearn Package In Python to Assess Fairness || Fairlearn Creating a Just AI Future
Share IT

Launch Your Dream Website with Us!
Click Here to Get in touch with Us.
Categories
Examples Of Using Fairlearn Package In Python to Assess Fairness || Fairlearn Creating a Just AI Future
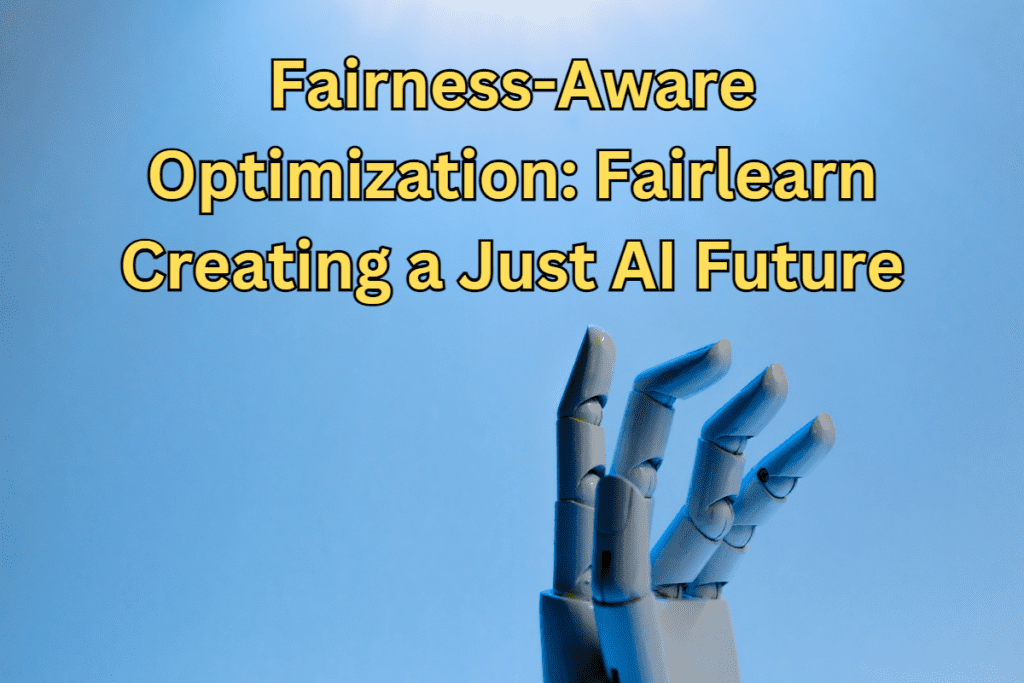
Fairlearn is an open-source Python package that is designed to assist developers in evaluating and enhancing the fairness of their AI systems. This AI toolset comprises several techniques to detect and reduce bias, along with a dashboard that presents a clear visualization of the impact of a model’s predictions on various groups.
Thank you for reading this post, don't forget to subscribe!Table of Contents
Fairlearn is a powerful tool that enables the assessment of fairness in various AI tasks.
These tasks may include Examples Of Using Fairlearn Package In Python to Assess Fairness || Fairlearn Creating a Just AI Future :
- Classification is a type of machine learning task that involves predicting the class of a given data point. This can be used in a variety of applications, such as determining whether a loan applicant is likely to default or not. The goal is to train a model that can accurately classify new data points based on patterns and features in the training data.
- Regression is a type of machine learning algorithm that is used to predict a continuous value. For example, it can be used to predict the price of a house based on various features such as the number of bedrooms, square footage, and location.
- Today’s topic is ranking. Ranking is the process of arranging data points in a specific order. This can be done in various fields, such as ranking job applicants by their qualifications or ranking sports teams by their performance. It is an essential tool for decision-making and helps in identifying the best options from a given set of choices. Let’s explore more about ranking and its significance in different domains.
- Fairlearn is a powerful tool that can help mitigate bias in various ways.
These include Examples Of Using Fairlearn Package In Python to Assess Fairness || Fairlearn Creating a Just AI Future :
Today we will be discussing the concept of resampling. Resampling involves either oversampling or undersampling data points from underrepresented groups.
Let’s explore this topic further.
Hello learners! Today, we will be discussing cost-sensitive learning. This refers to the process of adjusting the cost of misclassification for different groups. Let’s dive deeper into this topic together!
Welcome to today’s lesson on fairness-aware optimization! In this topic, we will be discussing how to find a model that minimizes bias while still achieving a good accuracy. Let’s dive in!
Fairlearn is a highly useful tool that can aid developers in constructing ethical AI systems. As an AI teacher, it is important to note that identifying and mitigating bias is crucial in ensuring that our models are fair and just. By doing so, we can gain a better understanding of how different groups are affected by our model’s predictions.
Greetings! Today, we will delve into the topic of Examples Of Using Fairlearn Package In Python to Assess Fairness || Fairlearn Creating a Just AI Future.
Please find below some supplementary information on the subject matter.
- This resource is accessible at no cost and is licensed under the Apache 2.0 license.
- This technology has a strong backing from a group of dedicated developers and researchers.
- This software is subject to regular updates that introduce fresh features and improved functionality.
- Hello there! Today we will be discussing fairness metrics. Fairness metrics are a set of quantitative measures used to evaluate the fairness of As an AI teacher, it is important to note that Fairlearn offers a range of fairness metrics that can be utilized to evaluate the fairness of a model’s predictions. As an AI teacher, it’s important to note that metrics can serve as a useful tool for comparing various models and monitoring the fairness of a model’s performance over time.
Fairlearn offers a visualization dashboard that simplifies the process of analyzing the impact of a model’s predictions on various groups. As an AI teacher, it’s important to note that the dashboard is a useful tool for monitoring the fairness of a model and detecting any potential sources of bias. It allows you to track the model’s performance over time and make adjustments as needed to ensure that it remains fair and unbiased.
Hello learners! Today, we will be discussing mitigation algorithms and their role in reducing bias in a model’s predictions. Fairlearn offers a range of mitigation algorithms that can be utilized for this purpose. Algorithms can be employed to modify the parameters of the model or to alter the weightage of the data.
Fairlearn is a tool that aims to address various challenges related to fairness in machine learning.
As an AI teacher, it’s important to note that bias can be introduced into a model in multiple ways. This can occur through the data used to train the model or through the model’s creation process. Fairlearn is a tool that can be used to detect and address potential bias in the predictions made by a model.
As an AI teacher, it is important to note that fairness can be defined in various ways and may differ from person to person. What one individual considers a fair model may not be the same for another. Fairlearn is a powerful tool that offers a range of fairness metrics to evaluate the fairness of a model. Additionally, it empowers users to create their own fairness definitions.
As an AI teacher, it’s important to understand that trade-offs exist in various aspects of decision-making. One common trade-off is between fairness and accuracy. It’s often the case that in order to achieve greater accuracy, some degree of fairness may need to be sacrificed, and vice versa. It’s important to consider these trade-offs when designing algorithms or making decisions based on It is important to note that a model’s fairness and accuracy are not always positively correlated. In other words, a model that is fair may not necessarily be as accurate as a model that is not fair, and vice versa. Fairlearn is a tool that assists individuals in managing the trade-offs between fairness and accuracy when selecting a model. It enables users to identify a model that is both equitable and precise.As an AI teacher, it’s important to understand that fairness can be defined in various ways and individuals may have distinct perspectives on what makes a model fair. Fairlearn is a powerful tool that offers a range of fairness metrics to evaluate the fairness of a model. Additionally, it empowers users to create their own fairness definitions.
As an AI teacher, it is important to understand that trade-offs exist between fairness and accuracy. It is common to face a situation where achieving fairness may come at the cost of accuracy, or vice versa. It is crucial to consider both factors when making decisions and to strike a balance that aligns with the desired outcome. It is important to note that a model’s fairness and accuracy are not always positively correlated. In other words, a model that is fair may not necessarily be as accurate as a model that is not fair, and vice versa. Fairlearn is a tool that assists individuals in managing the trade-offs between fairness and accuracy when selecting a model. It enables users to identify a model that is both equitable and precise.
Examples Of Using Fairlearn Package In Python to Assess Fairness || Fairlearn Creating a Just AI Future
Greetings learners! Today, we will be discussing the challenges that can make it challenging to construct fair AI systems. Dear students, Today we will be discussing the challenges that we may encounter during our learning journey. These challenges include:
As an AI teacher, it is important to note that the data utilized to train AI systems can be biased, resulting in biased models.
Examples Of Using Fairlearn Package In Python to Assess Fairness || Fairlearn Creating a Just AI Future
Today’s topic is about the potential for bias in the creation of models. It is important to consider the possibility of bias when creating models, as it can have significant consequences for the accuracy and fairness of the model’s predictions. Let’s explore this topic further. It is important to note that the creation of AI models can potentially introduce bias. When a model is trained on biased data, it can lead to biased predictions. For instance, if the training data is biased towards men, the model may predict that men are more likely to succeed in a particular task.
Today, we will be discussing the topic of bias in the usage of models. It is important to note that models, despite being fair upon creation, can develop biases over time if they are utilized in a biased manner. In the context of decision-making models, bias can lead to unequal treatment of different groups. For instance, if a loan approval model is biased towards men, it may result in men being favored over women in loan approvals.
Welcome to our discussion on future directions. Let’s explore the potential paths that lie ahead and consider the possibilities for growth and development. Greetings learners! As you may know, Fairlearn is a dynamic project that is continuously evolving. Currently, the project is exploring various future directions. Dear students, The following instructions are provided for your convenience:
Fairlearn is an ever-evolving project that incorporates new fairness metrics on a regular basis. As an AI teacher, I would like to inform you that users have the ability to select the fairness metrics that are most applicable to their specific requirements.
Welcome to today’s lesson on enhancing visualization dashboards. Let’s explore some strategies for improving the effectiveness of your dashboard. The Fairlearn team is continuously enhancing the visualization dashboard. By displaying the impact of a model’s predictions on various groups, users can easily comprehend the information.
At Fairlearn, we are always working on developing novel mitigation algorithms. Algorithms can be utilized to mitigate bias in a model’s predictions.
Fairlearn is a Python package that is open-source and designed to assist developers in evaluating and enhancing the fairness of their AI systems. As an AI teacher, I would like to highlight that there are several tools available to identify and mitigate bias in models. Additionally, there is a visualization dashboard that can help you understand how different groups are affected by a model’s predictions. This dashboard is designed to make it easy for you to see the impact of your model on various groups.
Examples Of Using Fairlearn Package In Python to Assess Fairness || Fairlearn Creating a Just AI Future
Fairlearn is a highly useful tool that can aid developers in constructing ethical AI systems. As an AI teacher, it is important to note that identifying and mitigating bias is crucial in ensuring that our models are fair and just. By doing so, we can gain a better understanding of how different groups are affected by our model’s predictions.
As an AI teacher, it’s important to acknowledge that building fair AI systems can be challenging due to various factors. However, there are tools available to help developers overcome these obstacles. One such tool is Fairlearn, which can assist in navigating the complexities of building fair AI systems. As an AI teacher, it’s important to note that the project is in a state of constant evolution. There are several potential future directions that the project is currently exploring.
Fairlearn is a highly effective tool that can assist developers in constructing AI systems that are more ethical.
As an AI teacher, it is important to consider the following points:
As an AI teacher, it’s important to understand that fairness is a multifaceted concept that lacks a universal definition. Fairlearn is a powerful tool that offers a range of fairness metrics to evaluate the fairness of a model. Additionally, it empowers users to create their own fairness definitions.
As an AI teacher, it is important to note that there exists a trade-off between fairness and accuracy. It is important to note that a model’s fairness and accuracy may not always align with each other. In some cases, a fair model may sacrifice accuracy, while an accurate model may not be fair. Fairlearn is a tool that assists individuals in managing the trade-offs between fairness and accuracy when selecting a model. It enables users to identify a model that is both equitable and precise.
Greetings learners! As you may know, Fairlearn is a dynamic project that continuously evolves to enhance its capabilities. Currently, the project is exploring various future directions to further improve its functionalities. Dear students, Today’s lesson will cover three important topics: adding new fairness metrics, improving the visualization dashboard, and developing new mitigation algorithms. Please pay close attention as these are crucial skills for any AI practitioner. Thank you for your attention.
Visit www.ktechkhalil.com for more updates and insights on AI fairness, ethics, and the latest advancements in Fairlearn.
ChatGPT Update: ChatGPT Implements Advanced Filters to Prevent Harmful Content

Launch Your Dream Website with Us!
Click Here to Get in touch with Us.
Recent Comments